Research Methods II
Module III
Outline
- Introductions
- Syllabus
- Assign presentations
- Experimental methods
Introductions
Course
Continuation of Seminar on Research Methods I
Room #513 (Tue 10:30-11:50)
Instructor
Benjamin Lind, PhD
blind@hse.ru *
Office #419 (Tue 18.10-20.10)
Students
*Introductions
Foreign instructor has a PhD, but no patronymic, how do we address him?!?
-
Preferred
- "Ben" or "Benjamin"
- Young, male sociologist from California--it's all good
-
Appropriate Formalities
- "Professor Lind," "Prof Lind," "Dr Lind"
- American-appropriate, yet not Russian-appropriate
-
Poor Formalities
- "Mr Lind"
Course
- Goal
- Build upon previous course
- Provide a comprehensive understanding of advanced and specialized research design strategies
- Instructional style
- American, conversational and informal
- Emphasis on interaction
- De-emphasis on physical documents
- Timing
- One week full-lecture
- One week odds and ends
- Language vow
Students
-
State your name
- Favorite methodological subject
- Last item bought
Syllabus
Syllabus
Click me for the syllabus
Click me to download the readings
Syllabus
-
Nine subjects
- Experiments, sampling, case studies, content analysis, secondary data, historical analyses, social network analysis, simulations, and synthesis
- Readings
- < 50 pages on subject
- General approach
- One general intro
- One empirical work
- Students present empirical work
Grading
- Attendance (15%)
- Participation (15%)
- Group presentation (15%)
- Review (3.75%)
- Evaluate (3.75%)
- Relate (3.75%)
- Dialog (3.75%)
- Assignments (15%)
- Final examination (40%)
Presentation Assignments
Readings
Salganik et al. (2006) [next week]
Rhomberg (2010)
Robinson (1976)
Barber (2001)
Armstrong and Crage (2006)
Christakis and Fowler (2007)
Morris and Kretzschmar (1997)
Random Groups of Three
Presentation Assignments
Presentation Assignments
Did I miss anyone?
Did I mistakenly include anyone?
Experiments

The following lesson relies upon and draws heavily from Neuman (2007), Chapter 8.
Experiments
Basic Troubleshooting Steps
-
Hypothesis
-
Modify situation
-
Compare with and without modification
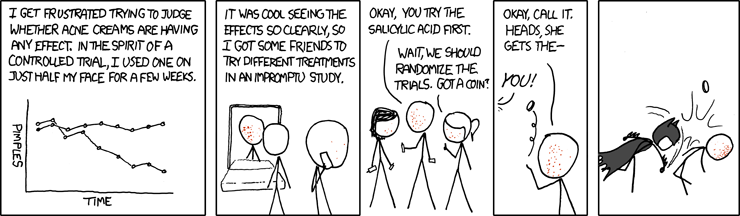
Experiments are the Best for a Causal Test
Causation requirements
- Temporal Order
- Association
- No Alternative Explanation
Experiments Require an Intervention
-
Which units of analysis would this requirement preclude?
- Which types of questions would it preclude due to issues of ethics and practicality?
E.g.,

E.g.,

Etc.

What are some ways we can intervene?
Random Assignment
- What is meant by random assignment?
- How are assignments determined?
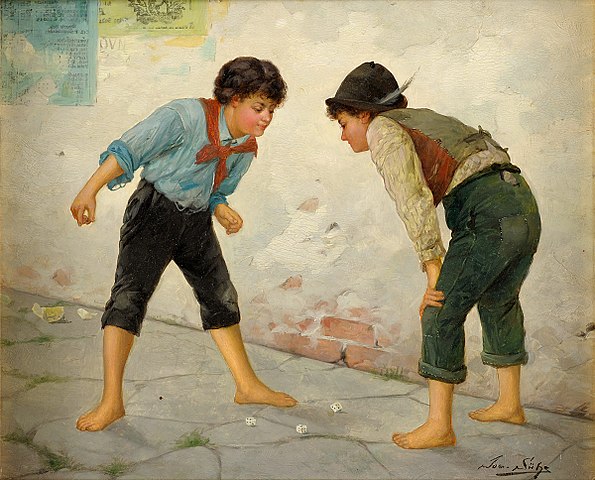
The Seven Parts to a True Experiment
- Random assignment
- Control group
- Experimental group
- Pretest
- Treatment / Independent variable
- Posttest
- Dependent variable
Why use a control?
To eliminate alternative explanations
Deception
- What are some examples?
- What are some ethical considerations?
- Role of debriefing subjects.
Types of Experimental Designs
Notation
- O = Dependent variable
- O1 = Pretest
- O2 = Posttest
- X = Treatment
- X1 = First treatment
- X2 = Second treatment
- ...
- R = Random assignment
- Rows represent groups
- Z = Confounding factor (factorial design)
Neuman (2007) Basics of Social Research, p. 213
Debates
- To pretest or not to pretest?
- Benefits of pretest
- Benefits of avoiding pretest
-
Which designs lends themselves to macro inquiry?
- How can we identify them?
- How strong is their causal claim?
Internal Validity
"[T]he ability to eliminate alternative explanations of the dependent variable" Neuman (2007:212)
- Selection bias (if no random assignment)
- Contamination between subjects
-
Historical circumstances
-
Testing (i.e., pretest effects)
-
Instrumentation (i.e., slow equipment failure)
- Experimenter effects
- Maturation
- "Mortality"
- Statistical regression
External Validity
"[T]he ability to generalize experimental findings to events and settings outside the experiment itself" Neuman (2007:216)
- Reactivity
- "Hawthorne effect"
- Subject awareness of experiment changes how they respond
- Field experiments
- Natural settings
- Verify external validity
- Greater generalization, but limited control
Assignment One
-
I will e-mail you a link to take an online survey
- Results will be kept confidential
- Please take it seriously
- I will use your responses throughout the course
- Please do not discuss it with your peers
- Fill it out in a private setting
- Do not talk to your classmates about its
- Questions
- Format
- Images
- You must complete it by Friday
Assignment One Debriefing
- What did the survey ask?
- What were the researcher's main interests?
- How did you know?
- Was the survey an experiment?
- Can one conduct an experiment with a survey?
- What leads you to believe it was or was not?
- If so, what was the treatment?
- Did any questions stand out as unusual?
- How did you recognize them as unusual?
- What do you think their purposes were?
Assignment One Debriefing
- Yes, it was an experiment
- Randomly selected 50% of students for treatment*
- Two e-mails, two mostly identical surveys
- Some questions were repeated.
- Examples?
- Why ask the same questions a few times?
- Reliability
- Pretest/Posttest
- Treatment must occur between them
- What was the treatment?
Assignment One Debriefing
- No, it was not about cats!
- Sociologists don't care about people buying cats
- Pretest/post test on that was a ruse
- Slightly interested in cultural consensus
- "Lenin Cat" and "Hitler Cat"
- Human emotion ascription
- "Can additional survey questions affect the number of peer nominations?"
Peer Nominations
Which questions?
What are they useful for?
Assignment One Debriefing
Theory: Reactivity
"Respondents might nominate a different number of peers if they are aware the researcher was scrutinizing their responses."
- Two-tailed hypothesis
- H0: No difference between the control and the treatment
- H1: Difference between the control and the treatment
- Privacy concerns -> fewer nominations
- Interest in appearing thorough -> more nominations
Assignment One Debriefing
Operationalizing Reactivity
-
"Over the past two weeks, have you..."
- "...gone on a river safari with a student from our class?"
- "...been enrolled in the same course with at least two students from our class?"
Assignment One Debriefing
Results
Change in Nominations
Fewer | Same | More | |||
Control | 1 | 6 | 3 | ||
Treatment | 3 | 5 | 3 |
- Did the treatment affect the posttest?
- What are the implications?
Assignment One Debriefing
Limitations and Problems
- All subjects had already received a reactivity treatment!
- Which question(s)?
- Are surveys even appropriate to test reactivity?
- Deception came later, not well-integrated
- Small sample and absolutely no external validity

Student Presentation
Matthew J. Salganik, Peter Sheridan Dodds, and Duncan J. Watts. 2006. "Experimental Study of Inequality and Unpredictability in an Artificial Cultural Market." Science 311:854-6.
Next Week: Sampling
Sampling

The following lesson relies upon and draws heavily from Neuman (2007), Chapter 6.
Materials
Computer Scripts
Fundamental Terms
Universe
"The broad class of units that are covered in a hypothesis. All the units to which the findings of a specific study might be generalized." (Neuman 2007)
Population
"The name for the large group of many cases from which a researcher draws a sample and which is usually stated in theoretical terms." (Neuman 2007)
Fundamental Terms
Sampling Frame
"A list of cases in a population, or the best approximation of it." (Neuman 2007)
Sample
"A smaller set of cases a researcher selects from a larger pool and generalizes to the population." (Neuman 2007)
Fundamental Terms
What are some examples of a...
-
...universe?
- ...population?
- ...sampling frame?
Using the data from the class survey, what is the...
- ...universe?
- ...population?
- ...sampling frame?
Other Terms
Sampling Ratio
The number of sampled cases divided by the size of the population they represent
Population Parameter
A characteristic of the population, typically estimated with statistics
Sampling Error
The difference between the measured parameter in a sample and the population parameter
Statistical Terms
Central Limit Theorem
As the number of random samples on a measurement increase, their average approaches the population parameter
Confidence Interval
An interval in which a research claims, with a given degree of certainty, includes the population parameter
Sampling Distribution
"A distribution created by drawing many random samples from the same population" (Neuman 2007)
The Law of Large Numbers

Probability vs Nonprobability Sampling
Is there a known probability of a case being selected?
Nonprobability Samples
Types
- Convenience Sampling
- Quota Sampling
- Purposive Sampling
- Sequential Sampling
- Deviant Case Design
- Snowball Sampling
Nonprobability Samples
Haphazard/Accidental/Convenience Sampling
Photo courtesy of Anneli Salo
Nonprobability Samples
Haphazard/Accidental/Convenience Sampling


Nonprobability Samples
Haphazard/Accidental/Convenience Sampling


Nonprobability Samples
Haphazard/Accidental/Convenience Sampling
Population parameter:
Mean number of communication partners
Convenience sample:
First five students to take the survey
source("http://pastebin.com/raw.php?i=BU2tycrK") #Loads data and libraries
pop.param <- mean(studentdat$commties) #Measures population
first.five <- which(studentdat$timerank %in% 1:5) #Samples
convien.param <- mean(studentdat$commties[first.five]) #Measures sample
cat("\n Population parameter = ", pop.param, ", Sample parameter = ", convien.param, "\n")
source("http://pastebin.com/raw.php?i=BU2tycrK") #Loads data and libraries
pop.param <- mean(studentdat$commties) #Measures population
first.five <- which(studentdat$timerank %in% 1:5) #Samples
convien.param <- mean(studentdat$commties[first.five]) #Measures sample
cat("\n Population parameter = ", pop.param, ", Sample parameter = ", convien.param, "\n")
Nonprobability Samples
Haphazard/Accidental/Convenience Sampling
NEVER!
EVER!
EVER!
Nonprobability Samples
Quota Sampling

Photo courtesy of BrianZim
Nonprobability Samples
Quota Sampling
Steps
- Determine categories
- Determine how many to sample from each category
- Sample haphazardly until quotas are met
What are the problems with this sampling method?
Nonprobability Samples
Quota Sampling Application
- Categories: Females and Males
- Quotas: Four women and one man
- Convenience: First to fill out survey
Population parameter:
Mean number of communication partners
Quota sample:
m <- sort(studentdat$timerank[which(studentdat$male)])[1] #First male time ranks
f <- sort(studentdat$timerank[which(!studentdat$male)])[1:4] #First four females time ranks
quota.samp <- which(studentdat$timerank %in% c(m, f)) #Samples
quota.param <- mean(studentdat$commties[quota.samp]) #Measures sample
cat("\n Population parameter = ", pop.param, ", Sample parameter = ", quota.param, "\n")
Nonprobability Samples
Purposive/Judgmental Sampling
When is it appropriate?
- Different information from unique cases
- Population is generally inaccessible
- More detailed information on a targeted group
Continues until data or research exhaustion
Nonprobability Samples
Purposive/Judgmental Sampling
Outliers: The Story of Success is a non-fiction book written by Malcolm Gladwell….. In Outliers, Gladwell examines the factors that contribute to high levels of success. To support his thesis, he examines the causes of why the majority of Canadian ice hockey players are born in the first few months of the calendar year, how Microsoft co-founder Bill Gates achieved his extreme wealth, how The Beatles became one of the most successful musical acts in human history.... Throughout the publication, Gladwell repeatedly mentions the "10,000-Hour Rule".... (Wikipedia)
Nonprobability Samples
Purposive/Judgmental Sampling
Variant: Sequential Sampling
Continues until no new information or sample diversity attained
Nonprobability Samples
Purposive/Judgmental Sampling
Variant: Deviant Case
("extreme" case)
To be discussed during our lessons on case studies.
Nonprobability Samples
Snowball Sampling
("network," "chain referral," or "reputational" sampling)
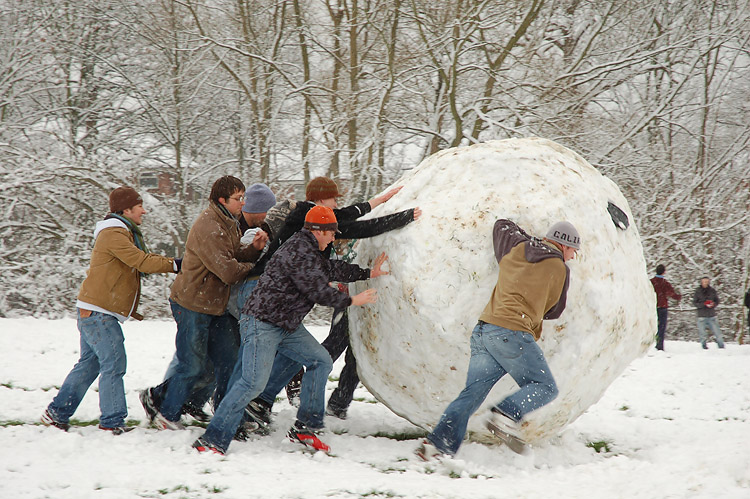
Nonprobability Samples
Snowball Sampling
Steps
-
Begin with seed(s)
-
Referrals from seed(s)
-
Sample referrals
Nonprobability Samples
Snowball Sampling

Nonprobability Samples
Snowball Sampling
Simulating the process
-
Start with seeds
-
Seeds refer peers
-
Peers might not respond
-
If peers respond, gain more referrals
-
Continue until sample size met or hit dead ends
Nonprobability Samples
Snowball Sampling
The Simulation Function
set.seed(666) #Standardizes random number generator
sb.samp <- function(n = 5, rr = .5, nseeds = 1, net = studentel){
recruit.wave <- rep(NA, times=vcount(studentel))
orig.seeds <- sample.int(nrow(studentdat), nseeds)
new.seeds <- orig.seeds
ncount <- nseeds
wave <- 1
recruit.wave[orig.seeds] <- wave
deadend <- FALSE
while ((ncount < n) & !deadend){
wave <- wave + 1
new.seeds <- unlist(sapply(new.seeds, neighbors, graph = net))
if (all(new.seeds %in% orig.seeds))
deadend <- TRUE
else{
new.seeds <- new.seeds[which(new.seeds %in% orig.seeds == FALSE)]
recruited <- which(rbinom(length(new.seeds), 1, rr)==1)
new.seeds <- new.seeds[recruited]
if ((length(recruited) == 0) | (length(new.seeds) == 0))
deadend <- TRUE
else{
orig.seeds <- c(orig.seeds, new.seeds)
recruit.wave[new.seeds] <- wave
ncount <- length(orig.seeds)
}
}
}
return(recruit.wave)
}
Nonprobability Samples
Snowball Sampling
Results
#General plot of "population" sociogram
library(RColorBrewer)
plot.wrap <- function(...)
plot(studentel, edge.width=7, vertex.label.family="sans", vertex.label.cex=1.25, vertex.label.dist=.85, vertex.label.degree=-1.9*pi/4, ...)
plot.wrap.sb <- function(sb.recruited){
sb.param <- mean(degree(studentel)[which(!is.na(sb.recruited))])
sb.maintitle <- "Snowball Sample Simulation"
sb.sub <- paste("Population Parameter = ", round(pop.param, 3), ", Sample parameter = ", round(sb.param, 3), sep="")
sb.recruits.color <- rev(brewer.pal(max(sb.recruited, na.rm=TRUE), "Blues"))[sb.recruited]
sb.recruits.color[is.na(sb.recruited)] <- "white"
plot.wrap(vertex.color = sb.recruits.color, main = sb.maintitle, sub = sb.sub)
}
plot.wrap.sb(sb.samp(5))
plot.wrap.sb(sb.samp(10, rr = .3, nseeds = 2))
plot.wrap.sb(sb.samp(10, rr = .8, nseeds = 2))
Nonprobability Samples
Snowball Sampling
Which social phenomena is this method good for studying?
Who are we more likely to reach in this population?
Who are we least likely to reach in this population?
Probability Samples
Types
- Simple Random Sampling
- Systematic Sampling
- Stratified Sampling
- Cluster Sampling
Probability Samples
Simple Random Sampling
Steps
- Acquire a reasonable sampling frame
- Determine sample size
- Randomly sample cases from the sampling frame
- Repeat until the sample size is met
- Sample without replacement
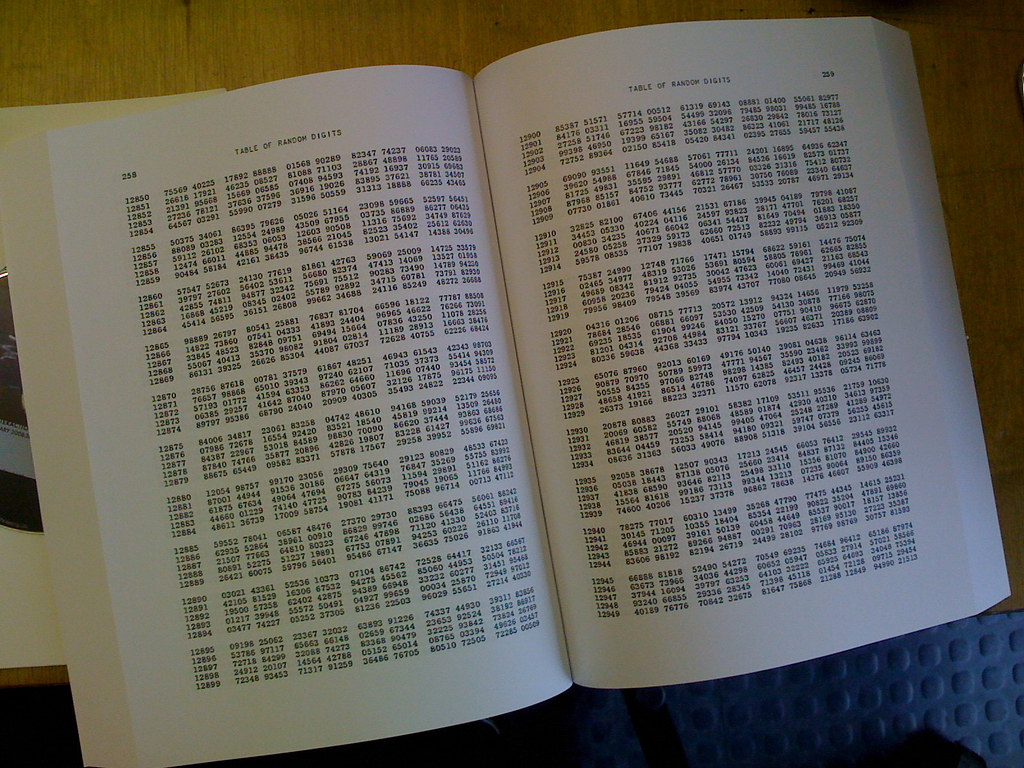
Photo courtesy of saschapohflepp
Webpages
Computer Programs
#Sample size of 10, ranging from 1 to 1000set.seed(666)
random.samp <- sample.int(1000, 10)
random.samp
random.samp.meanfun <- function(ss)
return(mean(studentdat$commties[sample.int(vcount(studentel), ss)]))
cat("\n Population parameter = ", pop.param, ", Sample parameter = ", round(random.samp.meanfun(5), digits = 3), "\n")
#To demonstrate the central limit theorem
random.mean.5samp.1k <- replicate(1000, random.samp.meanfun(5))
mean.across.randomsamples <- mean(random.mean.5samp.1k)
mean.across.randomsamples <- round(mean.across.randomsamples, digits = 3)
hist(random.mean.5samp.1k, main = "Frequency of Mean Communication Ties\nin 1000 Random Samples of 5", sub = paste("Population Parameter = ", pop.param, ", Mean Across Distributions = ", sep=""), xlab = "Mean Number of Communication Partners")
Probability Samples
Systematic Sampling
Steps
- Begin with a non-cyclical sampling frame
- Select a starting case at random
- Move up and down the list by every k cases
How would this method compare to random sampling?
How could a cyclical sampling frame affect your results?
Probability Samples
Systematic Sampling
# To demonstrate systematic samples
start.point <- sample.int(vcount(studentel), 1)
k <- 4
first.half <- seq(from = 1, to = start.point, by = k)
second.half <- seq(from = start.point, to = vcount(studentel), by = k)
syst.samp <- unique(c(first.half, second.half))
syst.samp
syst.samp.mean <- mean(studentdat$commties[syst.samp])
syst.samp.mean <- round(syst.samp.mean, digits = 3)
cat("\n Population parameter = ", pop.param, ", Sample parameter = ", syst.samp.mean, "\n")
Probability Samples
Stratified Sampling
Steps
- Identify mutually exclusive strata
- E.g., geographical units
- Randomly sample within each strata
- Weight to balance representation
Main benefits
-
Better representation than simple random sampling
- Why?
Probability Samples
Stratified Sampling
Consider our example "population"
(i.e., students in our class)
How could we construct a stratified sample?
Probability Samples
Cluster Sampling
(aka, "multistage sampling")
Steps
- Identify mutually exclusive strata
- Randomly sample strata
- Identify mutually exclusive strata within
- Randomly sample these strata
- Identify mutually exclusive strata within
- Randomly sample...
- Weight to balance representation
Probability Samples
Cluster Sampling
Consider our example "population"
(i.e., students in our class)
How could we construct a cluster sample?
Probability Samples
Cluster Sampling
Advantages
- Cost
- Speed
Disadvantages
- Less accurate than simple random sampling
- Requires detailed sampling frames
Tradeoff on cluster numbers and cluster size
Using the Telephone

Photo courtesy of Takkk
Using the Telephone
Is there a sampling frame?
-
Should you use it?
Random digit dialing as cluster sampling
-
What is it?
-
Why is it cluster sampling?
Words of caution
-
Role of telephones in social life
-
Nonresponse
-
Privacy
Weights
What do weights do?
Why are weights sometimes needed?
On which criteria should respondents be weighted?
Hidden Populations
What are hidden populations?
Capture-Recapture
Respondent-Driven Sampling
Scale-up Methods
Hidden Populations
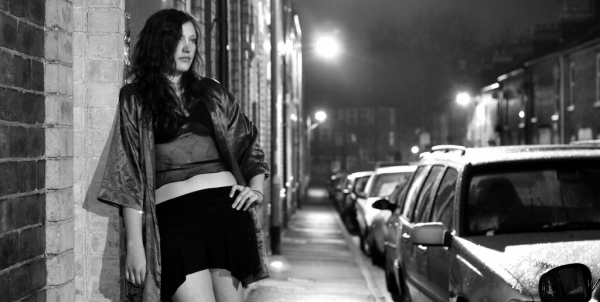
Photo courtesy of Oldmaison
Hidden Populations
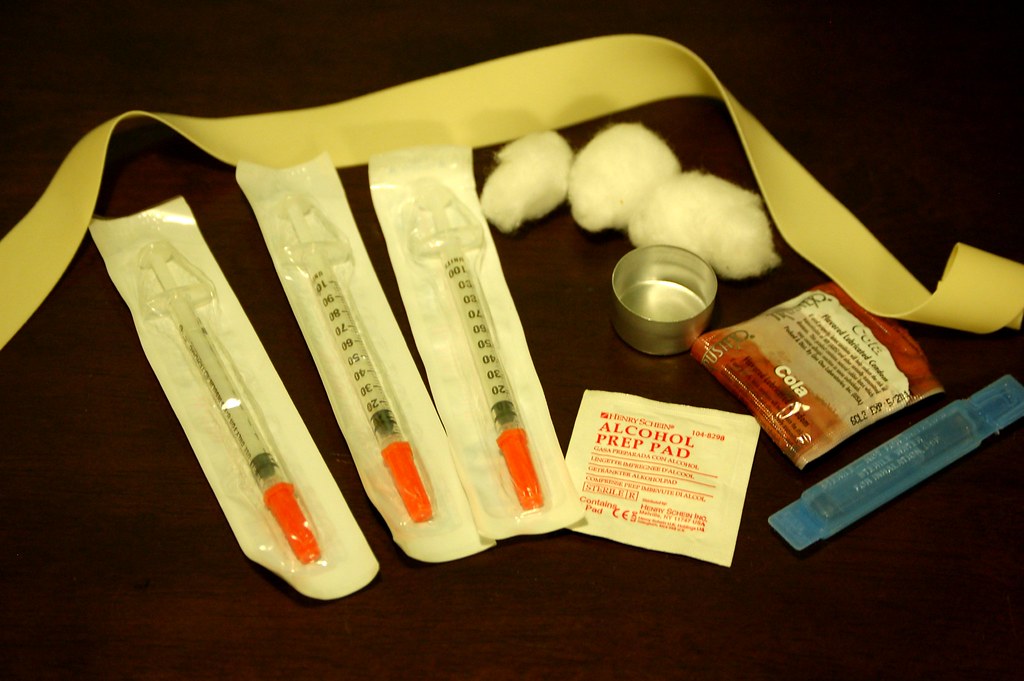
Photo courtesy of Todd Huffman
Hidden Populations
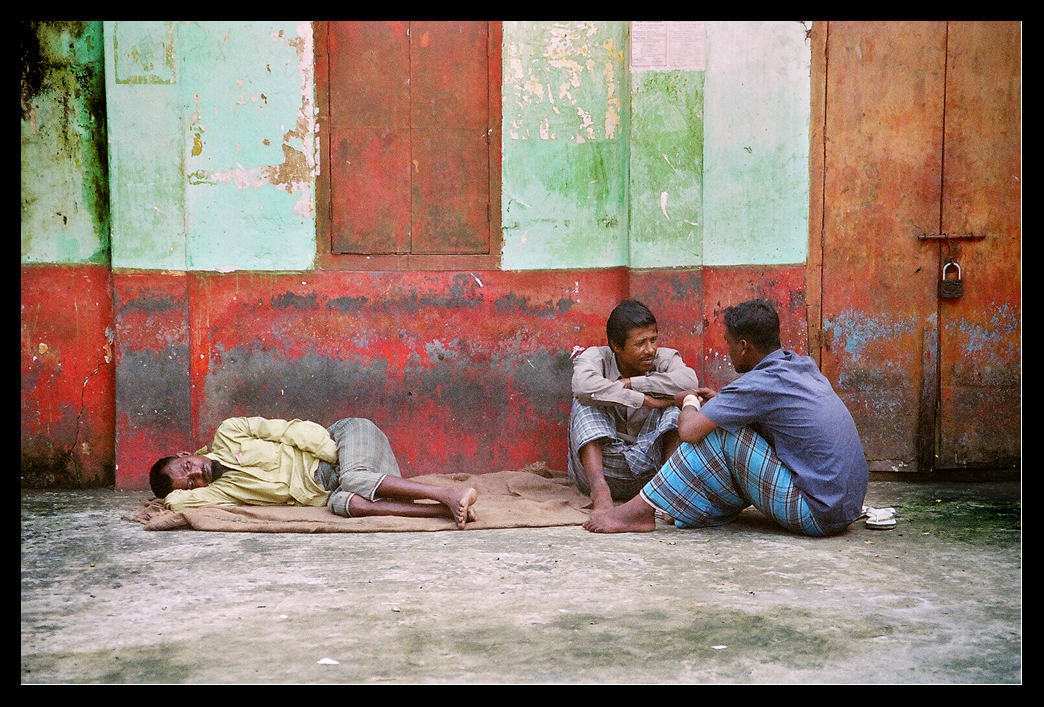
Photo courtesy of Orangeadnan
Hidden Populations
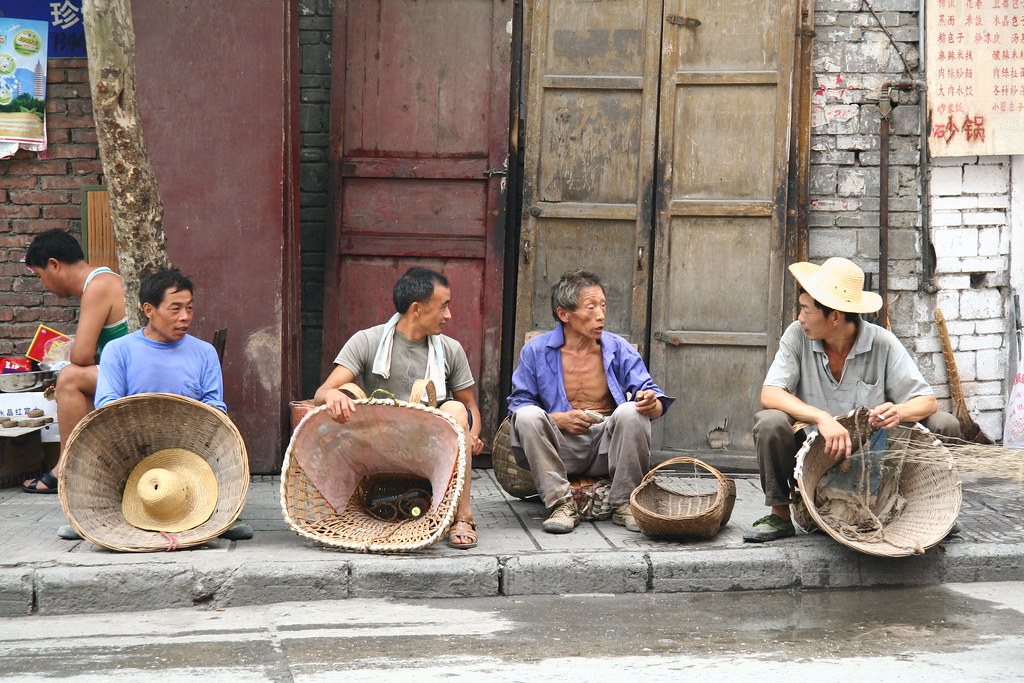
Photo courtesy of AdamCohn
Hidden Populations

Hidden Populations
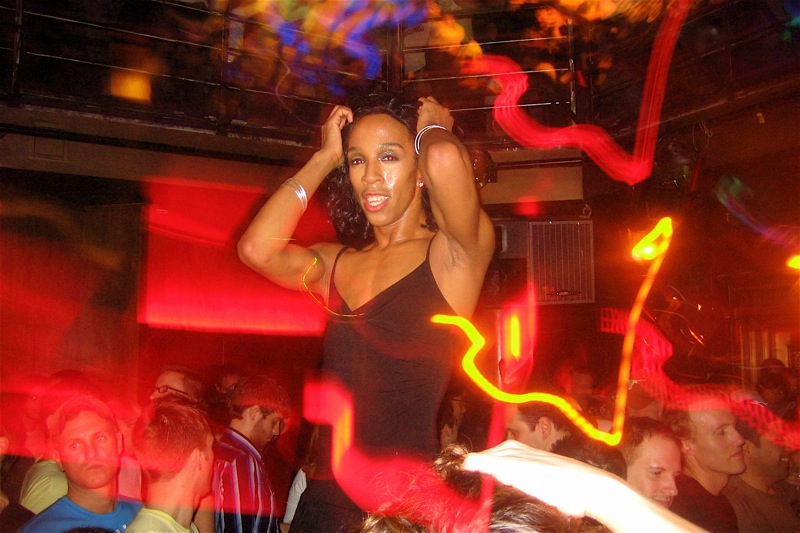
Photo courtesy of maxintosh
Hidden Populations

Photo courtesy of kargaltsev
Hidden Populations
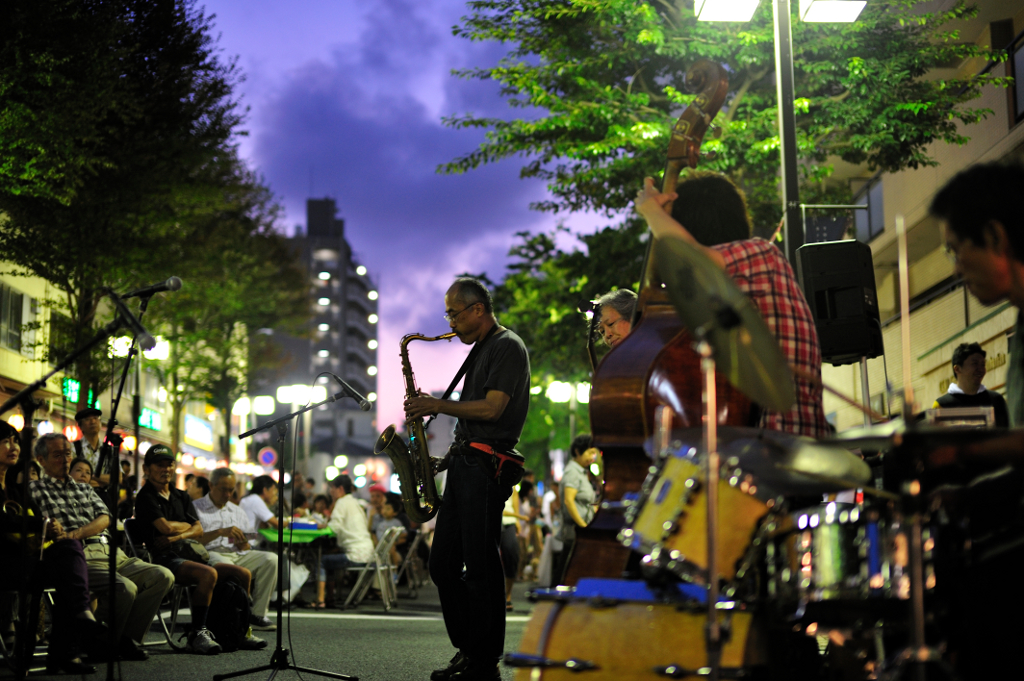
Photo courtesy of T-Hino
Hidden Populations
Lack a Sampling Frame
Characteristics
-
Population members interact with each other
- Isn't this true for all "populations?"
-
Often illegal or stigmatized, though not always
Commonalities: They're not Weberian Bureaucracies.
-
E.g., No clear organizational hierarchy or authority,
-
no written rules or neutral governing body,
-
no expert training, and
-
no meritocratic advancement
Hidden Populations
Capture-Recapture
Photo courtesy of Mickey Samuni-Blank
Hidden Populations
Capture-Recapture
Two Capture Sweeps
- M = First sweep, captured and marked
- R = Recaptured with marks
- C = All captured during second sweep
- N = Estimated total population size
N = M * C / R
R / M = C / N
How do we ethically "capture" and "mark" humans?
Hidden Populations
Scale-up Methods
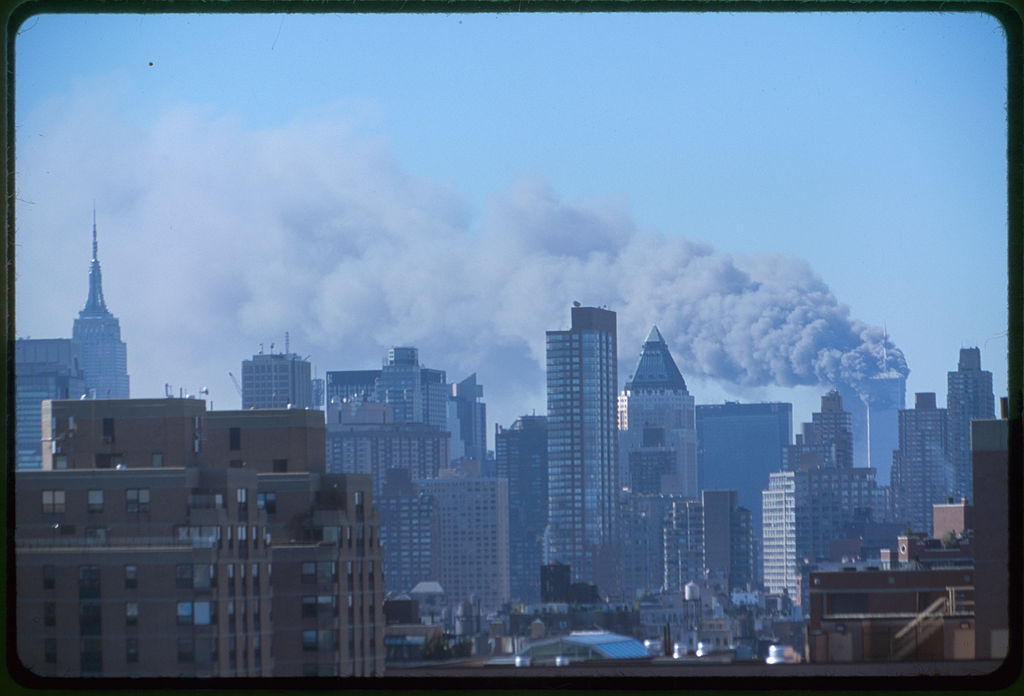
Hidden Populations
Scale-up Methods
- "How many incarcerated people do you know?"
- "How many licensed pilots do you know?"
- "How many people with the first name 'David' do you know?"
- ...
- "How many people do you know who died in the September 11, 2001 attacks?"
- Determine how many people respondent knows
- Extrapolate to a hidden population
Hidden Populations
Respondent Driven Sampling
(Heckathorn and Jeffri 2001)
- Location sampling
- Problem: Locations must be large & public
- Institutional samples
- Problem: Requires affiliation with institution
- Chain referrals ("snowball")
- Problem: Nonrandom "seeds"
- Problem: Volunteerism
- Problem: Differential recruitment
- Problem: Popularity effects
- Problem: Homophily and in-group effects
Hidden Populations
Respondent Driven Sampling
(Heckathorn and Jeffri 2001)
Address Problems of Chain Referrals
- Law of large numbers and Markov chains
- After enough waves, starting seeds don't matter
- Transition states and equilibrium
- Pay your respondents and make them comfortable
- Incentivize both the recruiter and the recruit
- E.g., Dropbox, plasma donation
- Use a limited number of "coupons"
- Respondents should come to the researcher
Hidden Populations
Respondent Driven Sampling
(Heckathorn and Jeffri 2001)
Address Problems of Chain Referrals
- Apply weights
- Understand who is likely/unlikely to be recruited
- Understand who is likely to recruit whom
- Homophilous recruitment as structure
- Recruitment is a behavioral network
- Can indicate communities and inequality
- Boundaries
- Screening process
Crowd Sampling
Photo courtesy of Roland zh
Crowd Sampling
Steps
- Understand event geography
- Break into teams
- Section crowd into geographical regions
- Interview every k person
- Record their responses
- Record basic information on refusals
Assignment Two
Next Week
Case Study Design
Case Studies

The following lesson relies upon and draws heavily from Gerring (2007)
Definitions
“Case connotes a spatially delimited phenomenon (a unit) observed at a single point in time or over some period of time.” Gerring (2007:19)
“A case study may be understood as the intensive study of a single case where the purpose of that study is -- at least in part -- to shed light on a larger class of cases (a population).” Gerring (2007:20)
Definitions
“At the point where the emphasis of a study shifts from the individual case to a sample of cases, we shall say that a study is cross-case.” Gerring (2007:20)
“An observation is the most basic element of any empirical endeavor.” Gerring (2007:20)
Typically, "
N
" refers to the number of observations
Population > Sample > Case ≥ Observation
Case Studies are Research Designs
- No prescribed data format
- No prescribed method of analysis
- No upper limits on the N
Neither inherently qualitative nor quantitative.
There are certain affinities, though.
Population typically difficult to discern.
Definitions
“A single observation may be understood as containing several dimensions, each of which may be measured ...as a variable.” Gerring (2007:20)
Y
- Dependent Variable
- Outcome of Interest
X
- Independent Variable
- Explanatory Variable/Factor
Data Organization
Data Frames and Matrices
- Rows represent observations
- Columns represent variables
- Grouping variable (could) represent cases
Typically done in a spreadsheet
Research Design Typology
Research must examine variation across cases or units
Dimensions of variation
- Number of cases
- One, "several," or "many"
- Form of variation
- Spatial and/or temporal
- Location of variation
- Within case and/or across cases
Research Design Typology
Why Case Studies?
Research Goals
Empirical Considerations
Case Study Research Goals
Tendencies
- Role of hypotheses
- Generating, rather than testing
- Needed to study new phenomena
- Validity
- Internal, rather than external
- Difficult to speak outside of sample
- Causality
- Focus on
- Mechanisms, rather than effects
- Inference
- Deep, rather than broad
Effects

Mechanisms

Griffin (1993:1110) AJS
Mechanisms

Griffin (1993:1110) AJS
Case Studies & Empirical Considerations
Tendencies
- Population of cases
- Heterogeneous, rather than homogeneous
- Causal relationship
- Strong, rather than weak
- Useful variation
- Rare, rather than common
- Data availability
- Concentrated, rather than disperse
Case Selection Strategies
-
Typical
- Diverse
- Extreme
- Deviant
- Crucial test
- Pathway
- Most-similar
- Most-different
Practical Reasons
- Language
- Data availability
- Theoretical background
Typical Case Selection
Representation
Hypothesis testing
Diverse Case Selection
Values range rather than distribution
Hypothesis testing
Hypothesis generation
Extreme Case Selection
Outliers
Representative only relative to larger sample of cases
Hypothesis generation
Deviant Case Selection
Outlier due to nonconforming relationship
Identify alternative relationships
Hypothesis generation
Crucial Test
Case least likely to exhibit relationship
- Explain Y
- Typical explanations X1, X2, X3, etc
- None explain Y for this case
- New variable, X4 works
Representativeness questionable
Hypothesis testing
Pathway
Select cases based on covariational patterns
(Combinations)
Interests
-
Mechanisms
-
Dependencies
Hypothesis testing
Most-Similar
Select very similar cases with different outcomes
Cases should have only one independent difference
That difference is the key variable
Maybe representative
Hypothesis testing and generating
Most-Different
Select very different cases with similar outcomes
Cases should have only one independent commonality
That commonality is the key variable
Maybe representative
Hypothesis testing and generating
Student Presentation
Chris Rhomberg. 2010. "A Signal Juncture: The Detroit Newspaper Strike and Post-Accord Labor Relations in the United States." American Journal of Sociology 115:6:1853-94.
Assignment 3
-
Break into five groups
- Each group is a case study design
- Create a "research design" with
- Unit of analysis
- Observation(s)
- Case(s)
- Outcome of interest, Y
- Explanatory factors, X1 and X2
Next week: Data entry and content analysis
Data Entry

Data Entry

Typical Ways to Enter Data
- Spreadsheet
- Text editor
- Automated
Demonstration
Let's create some simple data!
Typical Layout
- Units/Cases represented in rows
- Variables represented in columns
Delimited Text Files

- Advantages
- Cross-platform
- Easy to read
- Disadvantages
- Size
- Somewhat limited detail
Exercise
Without looking at your neighbor's responses, write down
No questions.
- Your name
- Your gender
- Your date of birth
- Your study group
- How many hours you spent studying this week
- How cats make you feel (Happy, Neutral, or Unhappy)
- One word to describe your class with Prof. Flores
- Who is your favorite character in Game of Thrones?
Now write it on the board, exactly as you wrote it on paper.
Typical Sociology Data Types
-
Boolean
- Numeric
- Integer vs. Real
- Date and Time
- Numeric, Text, Other Formats
- Ordinal
- Numeric
- Text
- Text
- Factors
- Descriptive
What are examples of each form of data type?
Missing Values

Missing Values
- Types of missingness
- Representation
- Handling
- Additional data (Best)
- Mean substitution (Bad)
- Case-wise deletion (Conventional)
- (Multiple) Imputation (Good)
- Random ("Hot deck")
- Nearest neighbor
- Estimation
Content Analysis

Much of the material from this lesson draws from
Krippendorff (2004) Content Analysis: An Introduction to Its Methodology
Content Analysis
How to Collect Data from Texts
What is a "text?"
What sort of projects is this method good for?
Advantages
- "Dead"
- Reliability
- Replication
What are some disadvantages?
Content Analysis
Steps
- Unitizing
- Sampling
- Recording/Coding
- Reducing data
- Inferring context
- Narration
Provide an example of a text.
Krippendorff (2004:83)
Unitizing
Which unit will you be recording?
Unitizing
"Even though large tracts of Europe and many old and famous states have fallen or may fall into the grip of the Gestapo and all the odious apparatus of Nazi rule, we shall not flag or fail. We shall go on to the end, we shall fight in France, we shall fight on the seas and oceans, we shall fight with growing confidence and growing strength in the air, we shall defend our Island whatever the cost may be, we shall fight on the beaches, we shall fight on the landing grounds, we shall fight in the fields and in the streets, we shall fight in the hills; we shall never surrender."How could this text be unitized?
Unitizing

Sampling
Which samples could these examples represent?
Which limitations would these samples face?
How could we sample within these examples?
Recording/Coding
Let's use a panel as our unit. What could we code?

Reducing Data
E.g.,
What proportion of panels portray violence?
What proportion of panels with violence display violence directed against one or more Nazis?
What proportion of nouns in Churchill's speech were first person plural?
Inferring Context
What would be the context for these two examples?
Which sociological topics do they speak to?
Narration
Natural Language Processing
- Object character recognition (OCR)
- Named entity recognition
- Relationship extraction
- Sentiment analysis
Suggested Application
Object Character Recognition

Images of text are not machine-readable
Software required to convert text images
(Always check for quality.)
Named Entity Recognition
"Latoya Ammons, from Gary Indiana, and her three children claimed to have been possessed by evil spirits....Recently, the priest who dealt with the actual exorcisms of this family, Rev. Michael Maginot has signed with Evergreen Media Holdings to make his account of the story into a movie."
"Latoya Ammons [person], from Gary Indiana [location], and her three children claimed to have been possessed by evil spirits....Recently, the priest who dealt with the actual exorcisms of this family, Rev. Michael Maginot [person] has signed with Evergreen Media Holdings [organization] to make his account of the story into a movie."Source: Exorcism in Gary Indiana by Wikinews
Named Entity Recognition
What are some possible uses for sociologists?
Which relationships do you think could be extracted?
Sentiment Analysis
What is the emotional state of the author?
<Words associated with happiness> :D
<Words associated with unhappiness> :'(
Positive values suggest happiness
Negative values suggest unhappiness
Sentiment Analysis
Remember when I asked you to describe yourself?
"I am a hockey player. My course paper is about migration. I am from Tomsk."Sentiment = 0
"I am happy to get the opportunity to study sociology in the honors group. I am conducting my course project with <peer>. Our topic is on economic sociology."Sentiment = 2.5
Sentiment Analysis
Let's test a hypothesis!
Female students tend to express more positive sentiments than male students.
- Data: Student self-descriptions
- Processing: Data Science Toolkit
- Analysis: Difference of Means, t-test
- Findings:
- Means: males = 0.22, females = 1.57
- Statistics
- t = 6.91***
- df = 12.53
- 95% confidence interval: [1.00, ∞)
Photo Analysis
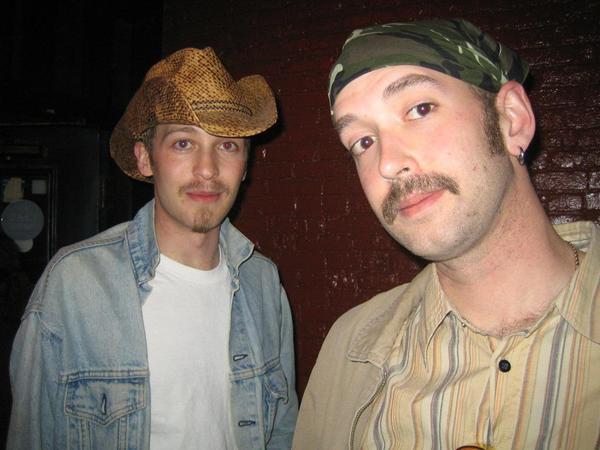
Can a computer detect...
- The number of people?
- Their gender?
- If they are smiling?
- If they have mustaches?
Photo by nosound
Photo Analysis
Application
Secondary Data

Data is all around us...
...and it's often free or cheap.
Secondary Data
Where to find it?
-
Libraries and electronic archives
- Statistical abstracts
-
Published articles
- Bibliographies
- Tables and figures
- Ask the authors
Libraries and Electronic Archives
Some examples
- ICPSR
- Archive.org
-
Datahub
- Quandl
- State released
Statistical Abstracts
Organized by subject
Offers description and reference to contemporary data
Publication of the Statistical Abstract of the United States stopped in 2012 due to budget cuts
Published Articles
Read the friggin' bibliography!
Andrews (2001:91)

Andrews (2001:92, 94-5)
Figures

McAdam (1983:739)
Figures

Marshakova, Irina V. 1981. Scientometrics 3, 1: 13-26.
Marshakova, Irina V. 1973. Scientific and Technical Information Serial of VINITI 6: 3-8
Figures
How would you convert a time series like McAdam (1983) into data?

Software
Ask the Authors
Their contact information is provided for a reason!
Reasons they say "no":
- Privacy
- Proprietary restrictions
- Future intentions with data
- Data lost
- Busy
-
Dishonesty
Reasons they say "yes":
- It helps science progress
- It strengthens the community
- Demonstrates honesty and finding integrity
Who creates and releases data?
Image courtesy of Nicknilov
Questions
What types of organizations are these?
What types of information do they release?
What the intended purposes for the data?
Typical units with public data?
Image courtesy of Brion VIBBER
Decades
...1900
1910
1920
1930
1940
1950
1960
1970
1980
1990
2000...
Years
2000
2001
2002
2003
2004
2005
2006
2007
2008
2009
2010
2011...
Divisions within a Year
Quarter 1
Quarter 2
Quarter 3
Quarter 4
Module I
Module II
Module III
Module IV
Months of the Year
January
February
March
April
May
June
July
August
September
October
November
December
What type of units are these?
Which fallacy are these units prone to?
Combining Datasets
"Mashups"
- Benefits
- Greater context
- Additional insight and contribution
- Often free
- Requires common identifier
- Geographical unit
- Time
- Industry, School, other organizations
- Doesn't require common unit of analysis
- Multilevel models
Limitations
- Have the analyses been done before?
- Is the data appropriate for the research question?
- Do you know the details of measurement and collection?
- Limited to the original variables and constructs.
- Are the items measured a proxy for your interests?
- No control over data collection.
- What information isn't collected?
- Administrative capacity.
Assignment
Challenge
Characteristics of the data behind the next diagram:
- All participants understood the data would be analyzed
- (Admittedly, for a different purpose)
- The data was collected unobtrusively
-
The data was reactive
- All members of our class are participants
- All members of our class
- Are capable of collecting it--no researcher privilege
- Have seen the original data--no privacy violation
- The data was free and quick to collect
- Data is a proxy measurement for "association"
Where did the data come from?
Implications
- Accuracy
- Behavioral
- Proxy requires researcher inference
-
Ethical
- Informed consent
- Anonymity
- Examples of related data?
- Building pass
- Assignment completion time
- Credit card purchases
- Metro rides
- ...
Historical Research

The following lesson relies upon and draws heavily from Neuman (2007), Chapter 12.
Content Analysis Assignment
- Two comics
- Similar books
- Subject matter
- Young, white female nurses
- Romance
- Audience
- Time: Oct - Dec & Oct 1961
- Different publishers
- Price
-
Comic Code Authority policy
Historical Circumstances
- "Golden Age" ~ 1938-1956
- 1954
- Comics Code Authority established
- Seduction of the Innocent
- "Silver Age" ~ 1956-1971
- Comics and gender
- Fear and anxiety
- Second Wave of Feminism ~ 1960s-1980s
- Feminine Mystique (1963)
Hypotheses
- Less gender equity in comics without CCA stamp
- Niche market and limited oversight
- (Not applicable for Dell Comics)
- Less gender equity in comics with CCA stamp
- Code recreate existing unequal discourse
- Code reduced creative outlet
- Emphasis on male-centric themes
- No difference in gender equity
- Non-objectionable content, no need for code
- Mass popularity
- Cultural regression to the mean
Operationalization
How did we measure gender equity?
Results
Assignment Questions
What was the case selection strategy?
What was the sampling strategy employed?
What are the suggested historical implications for gender socialization?
What are the other limitations to this exercise?
Historical Research:
Introduction
Purpose
- Challenge existing explanations and assumptions
- Expand subject of inquiry to new settings
- Specify or generalize
Difficulties
- Requires rich knowledge base on both
- Culture
- History
Steps
- Conceptualization
- Locating evidence
- Evaluating evidence
- Organizing evidence
- Synthesizing findings
- Narrative
Conceptualization
- Loose theoretical models
- Read some existent theoretical literature
- Imagine plausible models
- Background materials on case
- Encyclopedias
- Chronologies
- Generalist histories
Locating Evidence
- Bibliographies
- Additional literature
- Data sources
- Periodicals, reports, and white papers
- Datasets
- Archival materials
- Specialist libraries and archives
- Identify the important sites
- Locate pertinent and related materials
- Follow the archive's rules
- Record information and citation details
- Voice, notes, photocopies, scans, etc
Evaluating Evidence
-
Authenticity
- Original vs. secondary
- Assess probabilistically
- E.g., date created vs. date occurred
-
Reliability
- Internal and external consistency
- Literal vs. real meanings
- Created for researcher purposes?
-
Author's ability to be truthful
-
Socially able
-
Physically able
(Milligan, JD. [1979] History and Theory 18:2:177-96.)
Organizing Evidence
- Create a system of organization
- Spreadsheets
- Tagging systems
- Schemes
- Theoretical
- Chronological
- Case variation
Synthesis
How does your research fit into the existent literature?
Narration
Tell a compelling story for readers.
Evidence Types
- Primary Sources
- Secondary Sources
- Running Records
- Recollections
Primary Sources
Uses
- Originality
- Basis of historical knowledge
Downsides
- Laborious
- Often inaccuracy
- Biases
- Document retention
- Organization
- Literacy skills
Examples?
Example: Diaries

Northrop, John Worrell. 1904. Chronicles from the diary of a war prisoner in Andersonville and other military prisons of the South in 1864. Wichita, KS. p. 66.
Secondary Sources
Uses
- General understandings
- Broad descriptions
Downsides
- Subjectivity
- Selective inclusion/exclusion
- Causality
- Organization
- Narration
- Multiple and interactive effects
- Varying empirical strength
Running Records
Files and statistical documents produced by organizations.
Refer to the previous lesson.
Recollections
Individuals recounting their past experiences.
Uses
- Counteracts elite bias
- Absence of documentation
- People
- Activities
Downsides
- Accuracy and recollection
- Sensitivity
Example: Oral History

Comparative Research
Uses
- Wider range of observations
- Test cultural sensitivity behind theories
Downsides
- Laborious
- Sampling
- Limited generalizations
Units of Comparison
- Cultural regimes. Boundaries?
- Nation-states. Appropriate?
Comparative Research: Data
- Field research
- Primary
- Secondary
- Survey research
- Primary
- Secondary
- E.g., World Values Survey
- Content analysis
- Governmental statistical records
- Historical materials
Comparative Research:
Equivalence
Is the comparison appropriate?
Types of equivalence
- Lexical
- Contextual
- Conceptual
- Measurement
Social Network Analysis

Features
-
Intuition of social structure as ties bonding social actors
-
Informed by systematic empirical data
-
Visualization plays a substantial role
-
Requires mathematical and/or computational models
(Freeman 2004:3, 5)
What is a social network?
"A finite set or sets of actors and the relation or relations defined on them"
(Wasserman and Faust 1994:20)
What is an actor?
Actors are social entities
Actors do not necessarily have the ability to act
Actors (typically) are all of the same type
Formal terms for actors
-
Vertex
- Node
Examples?
Actors may also have attributes
(e.g., age, sex, ethnicity)
What are relations?
Social ties link pairs of actors
Relations collect a specific set of ties among group members
Related formal terms
-
Edges
-
Arcs
Conceptualizing Relations
- Directed undirected?
- Weighted or unweighted?
- Nominal, ordinal, interval, or ratio scale?
-
Signed or unsigned?
-
Loops?
-
Time sensitivity?
- Static
- Moving window
- Real-time
- Accumulation and decay
Relations may also have attributes
Two Basic Measurements
Degree
"Number of edges incident upon a node"
-
Undirected
-
Directed
- Indegree
- Outdegree
- Total (Freeman) Degree
Density
"Proportion of observed edges in a network"
Two Basic Measurements

Ways to Express a Social Network
- Sociogram

Ways to Express a Social Network
- Sociogram
- Matrix

Ways to Express a Social Network
- Sociogram
- Matrix
- Edgelist

Social Network Data
- Surveys
- Free response
- Roster
- Ego networks
- Field observations
- Documents
- Official reports
- Content analysis
- Published papers
- Websites
- Social media
- Links
Subgraphs
A set of nodes and edges within a graph
- Node-generated subgraphs
- Edge-generated subgraphs
Dyad Census
Dyad Census & Graph Properties
Undirected
-
Density (i.e., tie probability)
Directed
- Density (i.e., tie probability)
- Reciprocity

"You should attend funerals, because if you don't go to people's funerals, they won't go to yours."
Dyad Census & Graph Properties
Directed
- Density (i.e., tie probability)
-
Reciprocity
- Conceptual questions
- Are null ties reciprocal?
- Defined by edges or dyads?
- Common measurements
- Edgewise
- 2* M / (2* M + A )
- Dyadic
- ( M + N ) / ( M + A + N )
- Dyadic, non-null ("ratio")
- M / ( M + A )
Triad Census, Undirected
-
Brokerage
- Characterized by only two ties among three actors
- Transitivity, "clustering," triadic closure
- Your friends are often friends with each other
- Typically = (3*Triangles) / (Connected Triples)
Triad Census, Undirected

Triad Census, Directed
Triad Census, Directed
- Brokerage
- i → j → k , i ↛ k, k ↛ i
- Transitivity
- Weak (most common)
-
i
→
j
→
k, if
i
→ k
- Strong
- i → j → k, iff i → k
- Cycles
-
i
→
j
→
k
→
i
Walks
"A walk is a sequence of nodes and lines, starting and ending with nodes, in which each node is incident with the lines following and proceeding it in the sequence." - Wasserman and Faust (1994:105)
Trail
A walk such that every edge traversed is unique
(yet not necessarily every node
)
Path
A trail such that every vertex traversed is distinct
There could be zero, one, or multiple walks, trails, and paths between any two vertices!
Seven Bridges of Königsberg

Problem: Walk must cross every bridge only once
Euler (1735) proved there is no solution for the walk
-
Land masses are nodes, bridges are edges
- Would need zero or two nodes of odd degree
Measurements of Distance
Pairwise
Path length: Number of edges traversed between two nodes
Geodesic: Shortest path between two nodes
Geodesic distance: Length of the shortest path between two nodes
Graph and Subgraph
Average path length
Mean geodesic distance
Diameter: Longest geodesic distance
Measurements of Distance

Cycles
A walk "that begins and ends at the same node" and has "at least three nodes in which all lines are distinct, and all nodes except the beginning and ending node are distinct."
Wasserman and Faust (1994:107-8)
Cycles have a length
Connectivity and Components
If a path exists between each pair of vertices in a graph, then the graph is connected
- Strong connectivity: preserves path directionality
- Weak connectivity: ignores path directionality
A component is a maximally connected subgraph
An isolate is the smallest possible component: a single vertex without any ties to other vertexes in the graph
How many components?
Connectivity and Components
A bridge is an edge that, if removed, creates more components
A cutpoint is a node that, if removed, creates more components
Find Bridges and Cutpoints
Centrality and Centralization
Centrality: Nodal measurement
Who are the most important actors in a network?
Centralization: Graph measurement
How much difference in "importance" is there between actors within a network?
Generally, compares the observed network's centralization against the theoretical maximum
Centrality and Centralization
- Degree
- Betweenness
- Closeness
(Freeman 1979)
Betweenness
How many geodesics go through a node (or edge)?
Variations
Edge weighted
Edge betweenness
Proximity, Scale Long Paths, and Cutoff
Endpoints
Edge betweenness
Proximity, Scale Long Paths, and Cutoff
Endpoints
Random walk
Closeness
Q: What is closeness?
A: The inverse of farness!
Q: What is farness?
If connected, the sum of a node's geodesic distances to all other nodes
Variations:
Unconnected graphs
Edge weighted
Random walk
Cohesive Subgroups
“the forces holding the individuals within the groupings in which they are” - Moreno and Jennings (1937:137)
Cohesive groups tend to
- Interact relatively frequently
- Have strong, direct ties within themselves
- Display high internal density
- Share attitudes and behaviors within themselves
- Exert pressure and social norms internally
Cliques
A maximally complete subgroup - Luce and Perry (1949)
~In other words~
Everyone has a tie to everyone else in the subgroup (complete)
No other, smaller subgroups include only a subset of the same actors (maximal)
Critique: Too stingy!
4-cliques
3-cliques
2-Cliques
k-cores
Cohesive "seedbeds" nested within a network
Minimum #ties (k) each member of a subgroup has to other subgroup members
Directed graphs may measure
k
-cores through
- Ties going inward
- Ties going outward
- Total ties
Alvarez-Hamelin et al. (2006); Seidman (1983)
1-core
1 and 2-cores
1, 2, and 3-cores
Major Research Topics in Brief
- Homophily
- Diffusion
- Tie formation models
Homophily
("Assortativity")
Birds of a feather flock together
Homophily
Categorical vs. continuous variables
Sources?
Which relationships?
Felds's Foci
Forms of homophily
- Generalized
- Differential
- Matching
Intervening considerations
- Population effects
- Degree correlated attributes
- Triadic closure
Homophily
E-I Index
One (of many) measurements
EI = ( E - I ) / ( E + I )
E = #Ties between subunits
I = #Ties within subunits
Range: [-1, 1]
Lower values: More homophily
Higher values: Less homophily
Krackhardt (2003) The Journal of Applied Behavioral Science
Diffusion
The spread of a behavior or attribute
Diffusion
Requirements
- An artifact
- A sender
- A receiver
- A channel
Diffusion
Relationship to previous adopter increases a receiving node's propensity to adopt
Diffusion
Considerations
- Account for homophily
- Theorizing channels and artifacts
- What are some artifacts that could diffuse?
- Which channels could diffuse these artifacts?
- Conceptualizing time
- Adoption rate
- Decay
- Inhibitors
Modeling
How do ties form?
- Preferential attachment
- Homophily / assortativity
-
Block models
- Small world
- Other network evolution models
Preferential Attachment
-
Cumulative Advantage
-
Matthew Effect (Merton)
"For everyone who has will be given more, and he will have an abundance. Whoever does not have, even what he has will be taken from him." (Matthew 25:29)
-
Friendship Paradox (Feld 1991)
- Sensor research & epistemology
P(X=x) ~ x^(-alpha)
Nodes are of degree greater than or equal to
x
P
(X=x)
is the probability of observing a node with degree x or greater
alpha is the scalar
(Barabási and Albert 1999)
Blockmodels
Focus upon positions or "roles," not actors
Comprised of
- Discrete subsets of actors into "positions"
- Relationships within and between positions
Potential hypotheses
- Relationship between positions and attributes
- Structure of relationships
The following examples from Wasserman and Faust (1994:423)
Cohesive Subgroups
Center-periphery
Centralized
Hierarchy
Transitivity
Block Modeling
Which hypotheses do we have regarding the blocked structure of our class?
Time Point 1
Time Point 2
Time Point 3
Small World
Watts and Strogatz (1998)
Properties
- High clustering
- Short path lengths
Inspired by Milgram's "small world" experiment
Small World
Watts and Strogatz (1998)
- Begin with a ring-type graph ("lattice")
- Connect each node to k others
- Rewire (switch) the edges with β probability
Reality somewhere between
- "Connected caveman graph"
-
Random ties
Small World
Picture courtesy of Arpad Horvath
Other Evolutionary Models
Key questions as time proceeds:
- Actors
- Can they join a network?
-
If so, do they form a tie upon joining?
- Can they exit a network?
- Edges
- Can they form? Dissolve? Rewire?
- Mechanisms
- Under which circumstances do...
- Actors join or exit a network?
- Edges form, dissolve, or rewire?
- Actor-oriented or tie-based?
Other Evolutionary Models
References
- Robins and Pattison. 2001. "Random graph models for temporal processes in social networks." Journal of Mathematical Sociology, 25:5-41.
- Toivonen et al. 2009. "A comparative study of social network models: Network evolution models and nodal attribute models." Social Networks, 31:4:240-54.
- Snijders et al. 2010. "Introduction to stochastic actor-based models for network dynamics." Social Networks 32:1:44-60.
-
Krivitsky and Handcock. 2014. "A separable model for dynamic networks." Journal of the Royal Statistical Society, 76:1:29-46.
Separable Temporal Exponential Random Graph Models
(STERGM)
Modeling two separate processes
- Tie formation
- Tie dissolution
Constants
- Actors do not join or leave
- Actor attributes remain fixed
Data: Student communication networks
STERGM
Which hypotheses do you have regarding...
- tie formation?
- tie dissolution?
My hypotheses
- edges + group homophily
- triangles
- two-paths (dissolution only)
STERGM
-
Edges + Homophily
- Probability of a within group tie forming: 0.24
- Probability of a between group tie forming: 0.08
- Probability of a within group tie persisting: 0.80
- Probability of a between group tie persisting: 0.44
- Edges + Homophily + Triangle
- Tie formation
- No significant triangle effect
- Probability of a within group tie forming: 0.18
- Tie dissolution
- Probability of a triangle persisting: 0.71
- No significant homophily effect now
STERGM
- Differential models
- Formation: Edges + Homophily
- Dissolution: Edges + Triangles + Two-Paths
- Results
- Formation
- Probability of within group tie formation: 0.22
- Probability of between group tie formation: 0.08
- Dissolution
- Probability of a triangle persisting: 0.96
- Probability of a two-path persisting: 0.78
Observed vs. Simulated Networks
Exercise
Produce a research design with the following elements
- A research question
- A social network with clearly defined
- Actors
- Relationships
- Two hypotheses
- A means to test them with network
- Data
- Measurements
Simulations and Agent-Based Models

Why would a social scientist conduct computer simulations?
Ideas?
An Anecdote
FiveThirtyEight.com

An Anecdote
FiveThirtyEight.com
- Weighted Polling Average
- Adjusted Polling Average
- FiveThirtyEight Regression
- FiveThirtyEight Snapshot
- Election Day projection
- Error analysis
-
Simulation
Why would a social scientist conduct computer simulations?
Ideas?
- Methodological Robustness
-
Data limitations
- Measurement
- Model fit
- Theory
- Hypothesis testing
- Hypothesis generating
- Prediction
Data Limitations
What are some typical data problems?
(Assuming the operationalization matches the conceptualization.)
- Response rate issues
- Low response rate
- Unrepresentative respondents
- Missing data
- Error
- Respondent error
- Interviewer error
Response Rate Issues
Low response rates are often addressed with bootstrapping
Steps to bootstrap a measurement
- Calculate an initial measurement
- Resample within the responses with replacement
-
Recalculate the measurement
- Repeat Steps 2 and 3 many times (thousands)
- Construct a confidence interval
- Infer the population parameter
Missing Data
Why worry about it?
Techniques
- Case removal (default)
- Some problems, though...
- Imputation Methods
-
Additional data and inference (best)
-
Random value from within the data
- Completely at random
- Similar case
-
Model missingness and estimate
- Multiple imputation
- Impute -> Measure -> Repeat -> Combine
Error
How does respondent error enter a dataset?
How does interviewer error enter a dataset?

Error
Practically all data contain some error
Are your findings robust against it?
A way to find out:
- Take original measurement
- Introduce error to your data
-
Remeasure from error-riddled data
- Repeat Steps 2 and 3 many times
- Repeat Step 4, increasing the simulated error
- Compare simulated error to observed
Simulated Experiment
What are some forms of respondent error in our peer communication networks?
I propose the following peer selection error
- I intend to select <A>, but I instead select <B>
- Assumed constants
- Number of vertices, edges
- A student's degree
- Mistakes are random
Simulated Experiment
Let's focus on transitivity
-
For each time point we introduce more selection error
- Error rates: 5%, 10%, 15%, 20%, 25%
- At each time point and error rate we "rewire" edges
- "Rewiring" means edges randomly trade partners
- The proportion of edges rewired equals the error
- We measure transitivity using these faulty networks
- We repeat each step a thousand times
Results of the Simulation
Is transitivity on our networks robust against random selection error?
- Why or why not?
- Under which conditions?
Measurement
What if your measurement results from structural properties?
E.g., Is a transitivity score of .54 high or low?
It certainly depends upon both
- The number of vertices in a network
- The number of edges in a network
A Refresher
On our communication networks
- Transitivity decreased over time
- Number of edges increased
- Number of vertices stayed the same
- Homophilous networks
- Can interact with transitivity
Transitivity here is the proportion of closed two-paths
Let's Simulate a Homophily Network!
Constant parameters
- Number of nodes
- Density (approximately)
- Number of groups and their sizes
-
Density within and between groups
Varying parameters
- Random edge assignment, given above parameters
- Simulated networks modeled after each time point
One thousand simulations for each time point
Interpretation?
Model Fit
Does the model adequately produce the outcome of interest?
Linear Regression Equation
y = B * x + e
It expresses a relationship that can be simulated!
Tie formation (above) and dissolution (below)
Agent-Based Models
Exercises in Theory
Portions of the next few slides draw from Macy and Willer (2002)
Agent-Based Models
Four Common Assumptions
- Agents are autonomous decision makers
- Agents are interdependent and influence one another
- Agents follow simple behavioral rules
- Agents adaptive and look back to the past
Agent-Based Models
Simplicity and generality are key to good models.
Why is a particular model less theoretically useful?
Agent-Based Models
Two major questions
- Emergent structure, e.g.,
- Convergence vs. differentiation
- Influence and diffusion
- Emergent social order, e.g.,
- Adaptation from interactions
- Yields cooperation, trust, collective action
Agent-Based Models
Common explanatory factors
- Homophily
- Transitivity
- Density
- Relational stability (persistence)
- Reciprocity
Agent-Based Models
Quality models should
- Be simple
- Avoid relying upon biological metaphors
- Provide rigorous experiments
- Be robust to changes
- Exhibit external validity
- Test the validity of the field's claims
- Emphasize affects resulting from social factors
Benefits of Simulation Methods
- Cost
- Respondent cooperation
- Ethical
Limitations of Simulation Methods
- Often lacks of empirical data
- Assumptions and complexity reduction
- Or is it a benefit?
Assignment

Demonstration
- Review one network model
- Review one diffusion process
- Formulate three hypotheses
- Simulate network and diffusion process
- Evaluate hypotheses
One Network Model
Alexei Vazquez. 2003. "Growing Network with Local Rules: Preferential Attachment, Clustering Hierarchy, and Degree Correlations." Physical Review E 67, 056104
Network Growth
- Upon each turn
- Networks add a vertex (probability [1 - u])
- New vertex ties to random old vertex
- Potential ties created from new two-paths
- A random two-path closes (probability u)
- Turns continue until N vertices introduced
One Network Model
Vazquez (2003)
Parameters
- N: Number of vertices
- u: Density & transitivity
Properties
- Skewed degree distribution
- Transitivity
- Degree correlation
One Network Model
Vazquez (2003)
We're going to add one slight modification for added realism...
Randomly rewire edges with probability p.
Implications:
- Constant
- Degree distribution
- Density
- Number of vertices
- As p increases
- Transitivity decreases
- Number of components increases
One Diffusion Process
Mark Granovetter. 1978. "Threshold Models of Collective Behavior." American Journal of Sociology 83:6:1420-43.
Outcome of interest: binary decisions
Examples
- Innovation adoption
- Rumors and diseases
- Strikes
- Voting
- Educational attainment
- Leaving social occasions and migration
- Jumping onto the dance floor
One Diffusion Process
Mark Granovetter. 1978. "Threshold Models of Collective Behavior." American Journal of Sociology 83:6:1420-43.
Two main ideas
- Each Individual has a threshold
- A minimum proportion of people engaging in collective action required for the individual engage
- Varies across vertices from 0 to 1
- We'll be using a random uniform distribution
- Network ties can encourage or discourage engagement
- Granovetter says they count twice
- We'll be varying this constant
One Diffusion Process
Mark Granovetter. 1978. "Threshold Models of Collective Behavior." American Journal of Sociology 83:6:1420-43.
F(thresholdi, xit) = Decision of i to engage at time t
F(thresholdi, xit) = xit > thresholdi
xit = Engagedit / (Engagedit
+ Unengagedit )
Engagedit
= EngagedPeersit * (PeerEffect - 1) + AllEngagedt
Unengagedit
= UnengagedPeers
it
* (PeerEffect - 1) + Allunengaged
t
Example
- Network
- N = 100
- u = 0.35
- Rewiring probability, p, = 0.20
- Diffusion process
- Initially engaged = 5%
- Thresholds = random uniform distribution
- Peer effect = 2
- Number of trials = 5
Research Question
Given these theories on network tie formation and decision-making, why do some collective action episodes escalate more quickly than others?
Hypotheses
- Density and transitivity
- As density increases along with transitivity, the rate of collective action engagement will increase.
- Randomization
- As the proportion of rewired edges increases, transitivity will decrease and the number of components will increase, preventing collective action growth.
- Strength of peer effect
- Increasing the effect from one's network ties will increase the rate of collective action growth.
Our Simulation
Code available here:
Steps:
- Create a random network
- Use Vazquez model with N = 100 and u
- Randomly rewire edges with probability p
- Set thresholds with a random uniform distribution
- Set a random 5% of population as engaged
- Run through threshold model for five trials
- Measure average change from one trial to the next
- Repeat 100 times
- Regress effects on average change
Density and Transitivity Experiment
Parameters
- N = 100
- Rewiring probability (p) = 0.20
- Number of trials = 5
- Peer effect = 2
- Randomly vary u from 0 to 1
Findings
Estimate Std. Error t value Pr(>|t|)
(Intercept) 4.795 1.069 4.487 1.97e-05 ***
u 7.434 1.991 3.733 0.000317 ***
Multiple R-squared: 0.1245, Adjusted R-squared: 0.1156
Randomization Experiment
Parameters
- N = 100
- u = 0.35
-
Number of trials = 5
- Peer effect = 2
- Randomly vary rewiring probability, p, from 0 to 1
Findings
Estimate Std. Error t value Pr(>|t|)
(Intercept) 7.855 1.113 7.057 2.45e-10 ***
p -2.234 1.846 -1.210 0.229
Multiple R-squared: 0.01472, Adjusted R-squared: 0.004666
Both Experiments
Parameters
- N = 100
-
Number of trials = 5
- Peer effect = 2
- Randomly vary rewiring probability, p, from 0 to 1
- Randomly vary u from 0 to 1
Findings
Estimate Std. Error t value Pr(>|t|)
(Intercept) 6.594 1.294 5.094 1.73e-06 ***
u 3.880 1.651 2.350 0.0208 *
p -2.790 1.750 -1.595 0.1141
Multiple R-squared: 0.07746, Adjusted R-squared: 0.05844
Peer Effect Experiment
Parameters
- N = 100
- u = 0.35
- Rewiring probability (p) = 0.20
- Number of trials = 5
-
Randomly vary peer effect from 1 to 5
Findings
Estimate Std. Error t value Pr(>|t|)
(Intercept) 8.2278 1.5214 5.408 4.5e-07 ***
Peer Effect -0.4018 0.4621 -0.869 0.387
Multiple R-squared: 0.007653, Adjusted R-squared: -0.002473
Conclusions
-
We have simulated two processes
- Network tie formation
- Interdependent decision making
- We tested the "rate of engagement"
- Averages change over time in engagement
- We have confirmed one hypothesis and rejected two
- Confirmed: Density increases growth rate
- Rejected:
- Transitivity has no effect after controlling density
- Peer effect does not affect growth rate
- Somewhat contradictory finding
Discussion
How would you improve upon this model?
- Varying peer effect
- Across actors
- Over time
- Add and remove edges
Contemporary Challenges and Future Directions in Methods

Types of Challenges
Methods Guiding Theory
Every Day Methodological Proliferation
Methods Guiding Theory
Abbott. 1988. “Transcending Generalized Linear Reality.” Sociological Theory.
y = X * b + u
X(t) = X (t - 1) * B + U
Issues
- Fixed set of actors*
- Monotonic causal flow
- Univocal meaning*
- No sequential effects
- Casewise independence
- Context independent*
Methods Guiding Theory
Abbott. 1988. “Transcending Generalized Linear Reality.” Sociological Theory.
y = X * b + u
X(t) = X (t - 1) * B + U
Better Reality Models
- Demographic models
- Sequential models
- Network models
Everyday Methodological Proliferation
Savage and Burrows. 2007. “The Coming Crisis of Empirical Sociology." Sociology.
Savage and Burrows. 2009. “Some Further Reflections on the Coming Crisis of Empirical Sociology.” Sociology.
Sociologists no longer have a monopoly on social data.
Who collects the most data? How?
Anecdote
Target
Everyday Methodological Proliferation
Savage and Burrows. 2007. “The Coming Crisis of Empirical Sociology."
Sociology.
Savage and Burrows. 2009. “Some Further Reflections on the Coming Crisis of Empirical Sociology.”
Sociology.
Survey Problems
- Response rates
- Homogeneous units
- Commercial surveys
Related Search Terms

Everyday Methodological Proliferation
Savage and Burrows. 2007. “The Coming Crisis of Empirical Sociology."
Sociology.
Savage and Burrows. 2009. “Some Further Reflections on the Coming Crisis of Empirical Sociology.”
Sociology.
In-Depth Interview Problems
- Mastery outside of sociology
- Who?
- More commonplace
- Less interesting
Why conduct interviews when...
Individual-level Public Data
- Advantages
- Relatively accurate and detailed
- Behavioral
- Contextual, ripe for secondary data
- Education
- Occupation
- Geography
- Relationships
- Problems
- Selective sample
-
Selective information
- Same issues can be said of interviews
Demonstration
#NotAllMen / #YesAllWomen
Suggested Directions
- Continue to carefully construct good theories
- Collect data based upon its ability to address theory
- Case study designs
- Field research
- Historical cases
- Comparative cases
- Triangulate data sources
- Be very wary of sampling issues
- Select (and create) analytic methods according to theory
Example: Wiki Surveys
- Primary characteristics
- Greedy
- Collaborative
- Adaptive
- Pairwise comparisons
- Relative importance
- Establishes rank order with uncertainty modeled
Assignment
Seminar on Research Design II
By Benjamin Lind
Seminar on Research Design II
- 9,320